Adversarial learning aims to identify weaknesses in machine learning models. The goal is to identify potential problems that cannot be found using traditional evaluation using test sets. It has been used successfully in a wide range of applications, typically focused on a specific model or domain. In image classification, methods have been developed to fool models that recognize traffic signs by rather simple modifications of pictures. Another direction of adversarial learning aims to identify examples that could break or improve the training of the model if that example would be added to the training.
Our research is focusing on defending machine learning models from adversarial attacks. No defense is completely immune to adversarial examples. Most existing approaches are tied to a specific machine learning model and struggle to preserve good performance on clean inputs. As a result, few adversarial defenses have been deployed in real-world applications. Additionally, we are interested in the implications of adversarial machine learning on developing machine learning models, their training and evaluation, and their application.
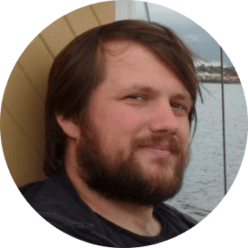
Joerg Simon Wicker
Gill Dobbie
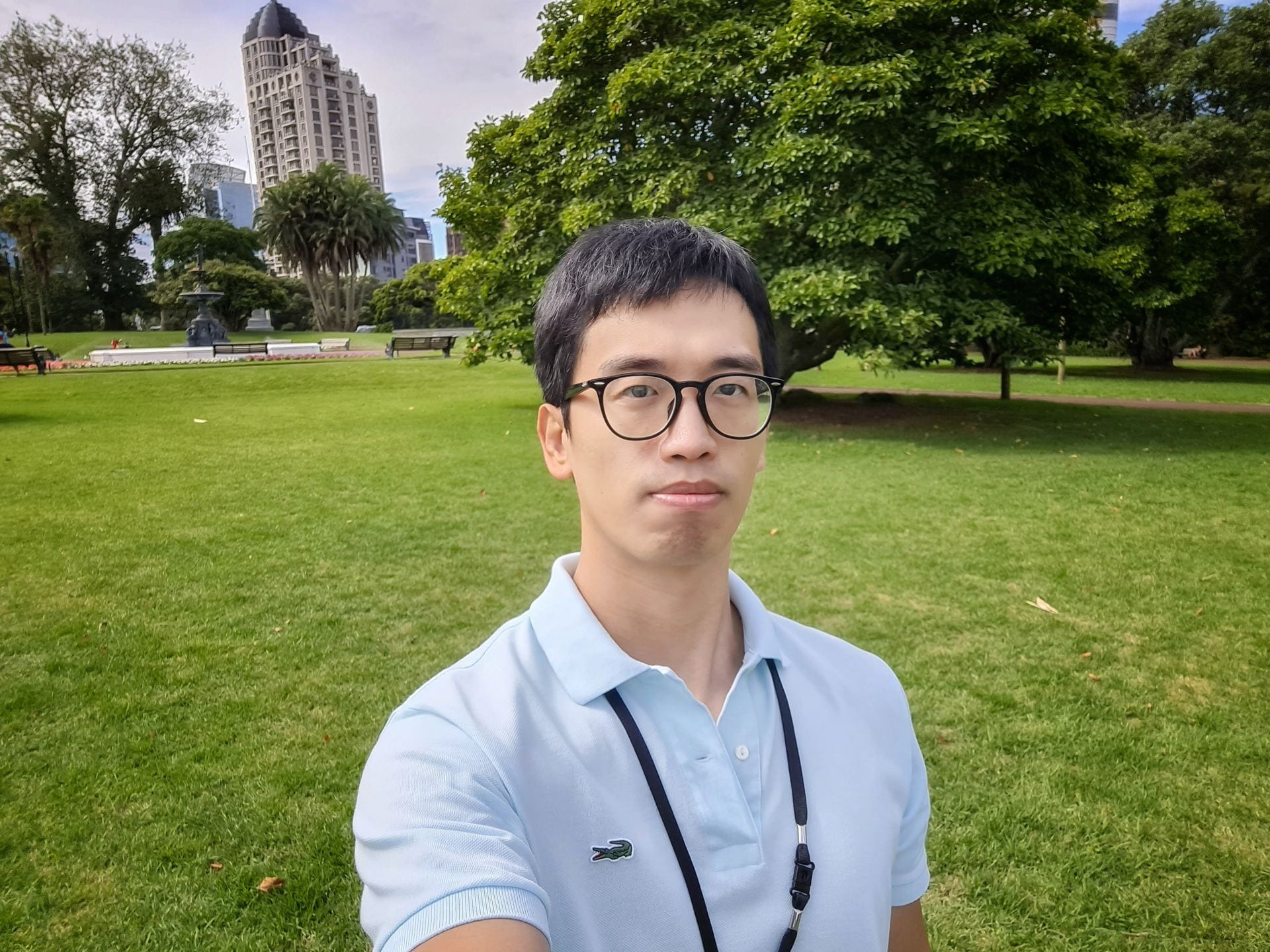
Luke Chang
Activities
Summer Project: Auditing Machine Learning Models – Quantifying Reliability using Adversarial Regions
Summary We aim to design and develop new methods to attack machine learning models and identify the lack of reliability, for example related to bias in the data and model. These issues can cause problems in various applications, caused by weak performances of...
AI Reading Group 06/10/21: Why Do Adversarial Attacks Transfer? Explaining Transferability of Evasion and Poisoning Attacks
Where and when: Thursday, June 10 at 2-3pm in 303S-561 Transferability captures the ability of an attack against a machine-learning model to be effective against a different, potentially unknown, model. Empirical evidence for transferability has been shown in...
AI Reading Group 15/Apr/2021: Adversarial Precision Sensing with Healthcare Application
For many real-world tasks obtaining a complete feature set is prohibitively expensive, especially in healthcare. Specifically, physicians must constantly balance the trade-off between predictive performance and cost for which features to observe. In this paper we...
Adversarial Learning: Robust and Reliable Machine Learning Models
Kia Ora! I am Luke Chang and I am passionate about building more reliable machine learning models, an artificial intelligence people can trust. I started my machine learning journey by designing a transmission controller for an engine in my third-year undergraduate...