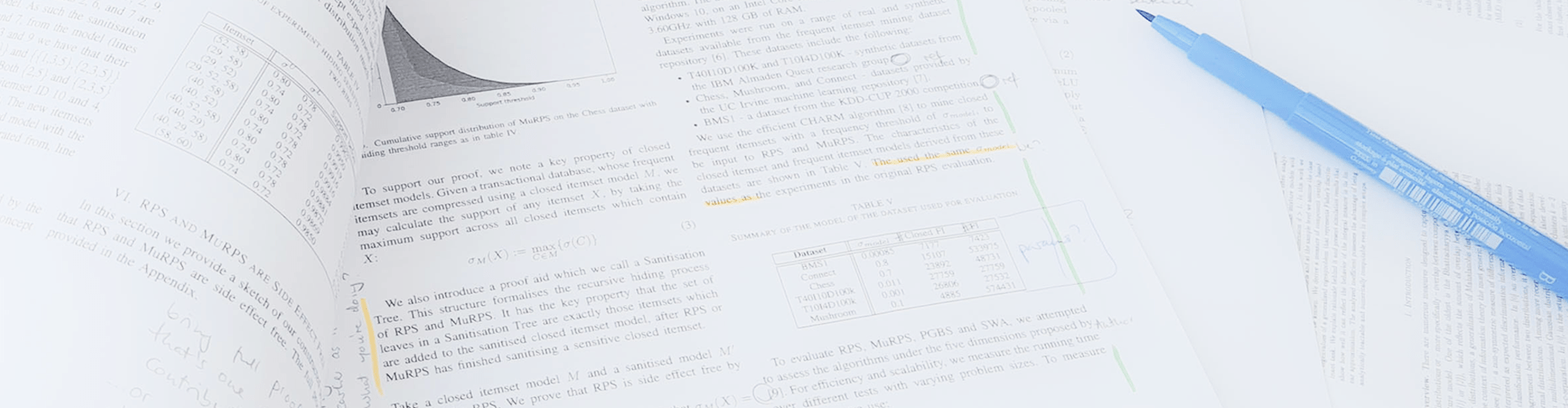
AI Reading Group
The AI Reading Group hosts bi-weekly reading groups where members present and discuss papers on topics in broad categories, such as AI ethics, machine learning, natural language processing, selection bias and computer vision. Come and join us in the discussion of ideas and seminal papers in AI Research to understand current developments and debates in the field!
The AI Reading Group will be held bi-weekly (alternating with ML Student Seminars) on Thursday 2-3pm in 303S-561.
Papers
Papers will be selected by alternating members of the group and the paper schedule will be announced two weeks in advance. We then encourage everyone to read the paper before joining the session. During each session, members will be discussing strengths/weaknesses/impact/novelty of the paper.
AI Reading Group 05/13/21: Active Learning Literature Survey
The key idea behind active learning is that a machine learning algorithm can achieve greater accuracy with fewer training labels if it is allowed to choose the data from which it learns. An active learner may pose queries, usually in the form of unlabeled data instances to be labeled by an oracle (e.g., a human annotator). Active learning is well-motivated in many modern machine learning problems, where unlabeled data may be abundant or easily obtained, but labels are difficult,...
AI Reading Group 04/29/21: Time-to-Event Prediction with Neural Networks and Cox Regression
New methods for time-to-event prediction are proposed by extending the Cox proportional hazards model with neural networks. Building on methodology from nested case-control studies, we propose a loss function that scales well to large data sets, and enables fitting of both proportional and non-proportional extensions of the Cox model. Through simulation studies, the proposed loss function is verified to be a good approximation for the Cox partial log-likelihood. The proposed methodology is...
AI Reading Group 15/Apr/2021: Adversarial Precision Sensing with Healthcare Application
For many real-world tasks obtaining a complete feature set is prohibitively expensive, especially in healthcare. Specifically, physicians must constantly balance the trade-off between predictive performance and cost for which features to observe. In this paper we tackle this problem by proposing precision sensing, formulating it as a time-dependent resource distribution problem described by a two-player minimax game between a dynamic feature sensor (FS) and a progressive learner (PL). FS...
Organisers
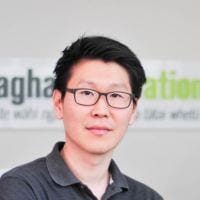
Jonathan Kim
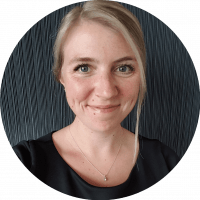