Short Bio
Dragi Kocev is a senior researcher at the Department of Knowledge Technologies, JSI and the CEO and co-founder of Bias Variance Labs. He completed his PhD in 2011 at the JSI Postgraduate School in Ljubljana on the topic of learning ensemble models for predicting structured outputs. He was a visiting research fellow at the University of Bari, Italy in 2014/2015. He has participated in several national Slovenian projects, the EU funded projects IQ, PHAGOSYS and HBP. He was co-coordinator of the FP7 FET Open project MAESTRA. He is currently the principal investigator of two ESA funded projects: GALAXAI – Machine learning for spacecraft operation and AiTLAS – AI prototyping environment for EO. He has been member of the PC of premium AI/ML conferences (e.g., DS, ECML PKDD, AAAI, IJCAI, KDD) and member of the editorial board of Data Mining and Knowledge Discovery, and Ecological Informatics. He served as PC co-chair for DS 2014 and Journal track co-chair for ECML PKDD 2017.
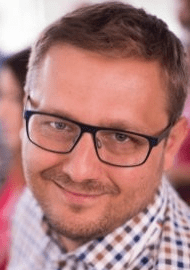
Abstract
Mars Express (MEX) is a spacecraft operated by the European Space Agency (ESA). It has been orbiting Mars since the beginning of 2004, providing a wealth of scientific invaluable data about Mars such as evidence of the presence of water on and below the surface of the planet, three-dimensional renders of the surface as well as data about the chemical composition of the Martian atmosphere. The scientific payload of MEX consists of a suite of seven instruments that provide global coverage of the planet’s surface, subsurface and atmosphere. The instruments andon-board equipment must be kept within their operating temperature ranges, spanning from +50◦C to temperatures as low as −180◦C. In order to maintain these predefined operating temperatures, the spacecraft is equipped with an autonomous thermal control system composed of 33 heater lines as well as coolers.
The thermal system, that maintains the temperature of the on-board components, consumes a significant amount of the total available electric power, leaving a fraction to be used for science operations. After more than 16 years in space, MEX’s components are slowly decaying, leading to reduced functionality and ever decreasing remaining lifetime, e.g., the batteries are now seriously degraded, making accurate planning and use of the available power essential. To this end, we use explainable methods for structured output prediction to estimate the thermal power consumption of MEX as accurately as possible. The thermal power consumption is estimated under a variety of operating conditions, including gyro-less flying and limited data availability. The used machine learning methods are from the framework of predictive clustering and consist of tree-based ensembles. Besides providing state-of-the-art predictive performance, the methods also facilitate explainability and understanding of the provided estimates.
The talk will be online via Zoom, to register, please submit the form below and we will send you the meeting link.