Short Bio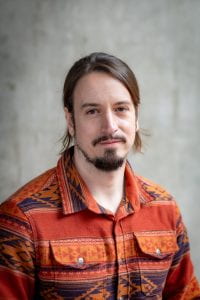
Pablo Montero-Manso is a Senior Lecturer at the University of Sydney, currently a Visiting Faculty Researcher at Google. He researches Machine Learning, Artificial Intelligence and Statistical tools for time series analysis, focusing on Forecasting, Classification, Clustering and Visualization of time series. Pablo has developed predictive models that resulted in award-winning performance in major forecasting competitions (both the M4 and M6) and have been adopted by industry. During the COVID-19 pandemic, he contributed with highly accurate models and predictions of the evolution of the pandemic that were part of the decision-making process in Australia, Spain and the European Union. Pablo is a member of the advisory board of the WHY project, analysing energy consumption of European households for policy making. He is an author and contributor to several open-source tools and open datasets in python and R, including the popular TSclust package for time series clustering.
Abstract
Pre-training machine learning models on large quantities of data has revolutionized many fields, but its adoption in Time Series Forecasting lags. It can be argued that fields such as Time Series Analysis, Signal Processing or Econometrics, have already produced very competitive models for time series that are difficult to outperform. In fact, the most successful neural network approaches to forecasting impose some form of structure derived from these ‘classic’ models.
We propose pre-training neural networks on trillions of data points coming from simulations of time series models, then run them in a zero-shot fashion on new time series. Surprisingly, these pre-trained networks can outperform the very model families that generated the data. Our research suggests they learn the underlying optimal estimation principles. For example, they are more accurate than a Least Squares or Maximum Likelihood estimator for an ARIMA model when the time series has been generated by an ARIMA.
An immediate benefit of this result is that pre-trained models can be deployed in place of existing forecasting solutions based on known processes, delivering accuracy gains at orders of magnitude faster compute time (even when run on CPU). More importantly, the method can approximately solve forecasting problems that have remained open and puzzled the forecasting community for decades, such as the notorious Optimal Forecast Model Selection/Combination, or derivation of good estimators for time series with complex uncertainty structures (mixtures, varying volatility, extreme events) or with structural breaks. It turns out that these processes are difficult to solve analytically, but trivial to simulate from.
These pre-trained models, after being trained on several orders of magnitude more data than other time series foundation models, achieve state-of-the-art in some of the main forecasting benchmark datasets already off-the-shelf, and further benefit from fine-tuning. We will end with a discussion of interesting challenges that arise from training via simulations: what to do when we have ‘infinite data’?
ML Seminar Room on Newmarket Campus: 903-414 in Building 903 (Level 4)
Webinar Link: https://auckland.zoom.us/s/92026566885